Theme 3
Effective Computer Vision for Conservation
The doctoral projects under Theme 3 have two main objectives.
Develop methodologies for vision-based drone control, enabling real time tracking, pose estimation and assessment of animal biometrics
Assessment of animal behaviour (which is at the core of Theme 1) requires the precise delineation of animal movement trajectories of herds, i.e., multiple animal tracking, but also the obtention of precise biometric characteristic of animals (posture, size, etc.). To obtain this information, being able to control the drone flight plan in almost real time is essential. But to be able to change the flight plan according to specific animals and remarkable features of interest being observed, an onboard vision system is required, so that the drone can fly closer to an animal to take the necessary images for identification and biometrics (DC12 – Adaptive Tracking). This way, the end user receives the images that are needed rather than those that are given by a pre-defined flight plan. Once these images (close ups, tracking of specific individuals showing characteristics of interest) are obtained, one can work on detecting animal biometrics such as animal posture, size, or its level of alert (DC9 – Individual Characteristics).
Develop principles and techniques for large-scale censuses of animal groups in their environmental context
Groups of wildlife move and interact in diverse and complex ecosystems. Conservation efforts require knowledge of the numbers and location of animals, the structure of their environment and the interaction of individual animals with their habitat. Thus, effective conservation drone systems require automation for large-scale assessment of animal locations and numbers (censuses). Currently, drone-based animal censuses are predominantly carried out through manual photo interpretation, which is costly, time-consuming, and challenging, in part due to the heterogeneous distribution of animals on the landscape and high terrain variability in aerial images[1]. Computer vision approaches aiming at automating censuses across geographical areas are urgently needed, as well a community engagement and software tools[2] for providing label information to train such models, information that can be obtained by citizen science and crowdsourcing[3] (DC11 – Interactive Census). Being able to detect, count and characterize animals (e.g., by their species) is a significant step towards population modelling that can then be used to study the interactions of animals. Since these behaviours are heavily influenced by the environmental context, we will extract high-precision reconstructions and semantic segmentations from the drone video data via detailed 3D modelling of the habitats (DC10 – Habitat Reconstruction).
References
Objective 2
[1] B.Kellenberger, D.Marcos, D.Tuia. 2018. Detecting mammals in UAV images: Best practices to address a substantially imbalanced dataset with deep learning. Remote Sens Environ, 216, 139-153.
[2] B.Kellenberger,D.Tuia,D.Morris. 2020. AIDE: Accelerating image-based ecological surveys with artificial intelligence. Methods Ecol Evol, 11, 1716–1727.
[3] F.Ofli, P.Meier, M.Imran, C.Castillo, D.Tuia, N.Rey, J.Briant, P.Millet, F.Reinhard, M.Parkan, and S.Joost. 2016. Combining human computing and machine learning to make sense of big (aerial) data for disaster response. Big Data, 4, 47–59.
Get in touch
Contact us on WildDrone@sdu.dk
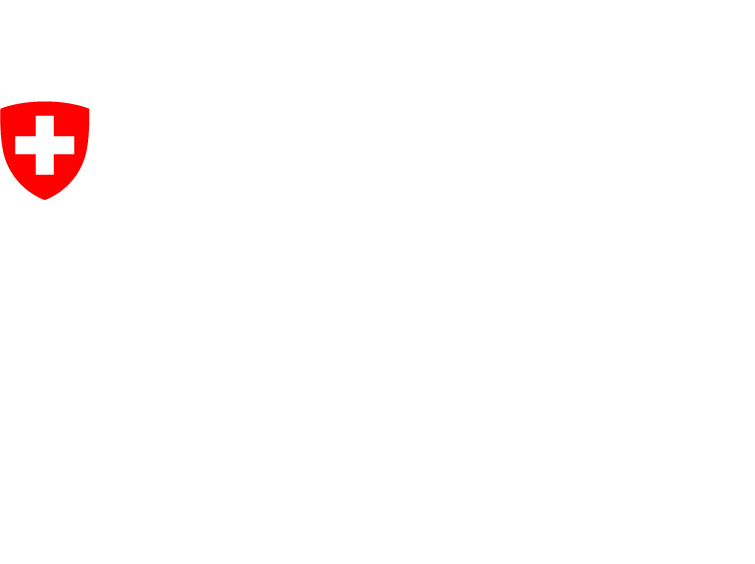
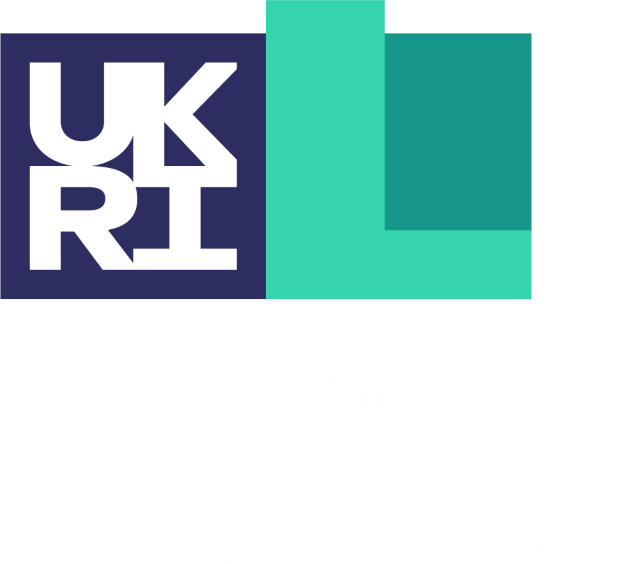
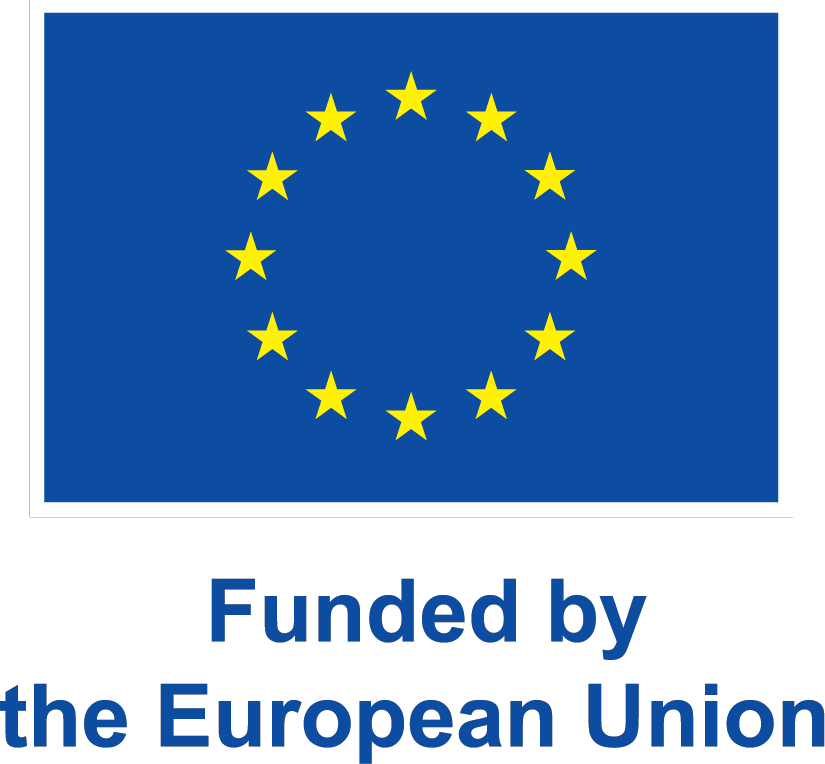
WildDrone is an MSCA Doctoral Network funded by the European Union’s Horizon Europe research and innovation funding programme under the Marie Skłodowska-Curie grant agreement no. 101071224. Views and opinions expressed are those of the author(s) only and do not necessarily reflect those of the European Union or the European Commission. Neither the EU nor the EC can be held responsible for them.