Habitat Reconstruction
Reconstructing Natural Habitats from Multimodal Drone Measurements
State of the Art
Most terrain reconstruction techniques for drone videos either use incremental or global optimization strategies to extract the 3D topology from 2D video footage (called photogrammetry, SfM, or SLAM). Each of these approaches is a trade-off between real-time capability and accuracy . However, since drone recordings in the wildlife context cannot guarantee optimal flight paths (e.g., revisits) drone-based SfM suffers from critical configurations, which are induced by ambiguities within the data . Furthermore, 3D reconstructions of organic structures, e.g., trees and plants can result in unnatural and bulky mesh models.
Innovations and Impact
An optimised 3D reconstruction and segmentation algorithm using drone video recordings to calculate accurate environmental reconstructions. It will deploy advanced graphics accelerations to make the model scalable to large natural areas. This process relies on multiple viewpoints of the area being observed. This can be obtained by exploiting the video footage recorded by drones and the spatiotemporal overlaps. Overall, this leads to accurate characterization of the morphology of animal habitats.
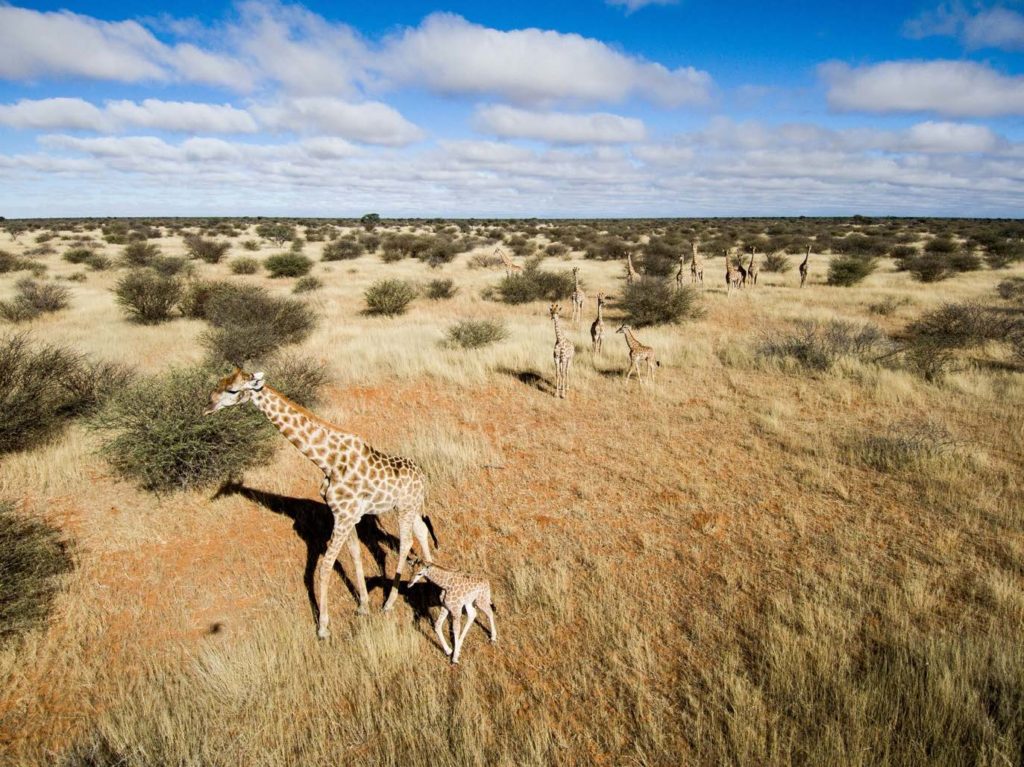
Copyright by Kuzikus Wildlife Reserve
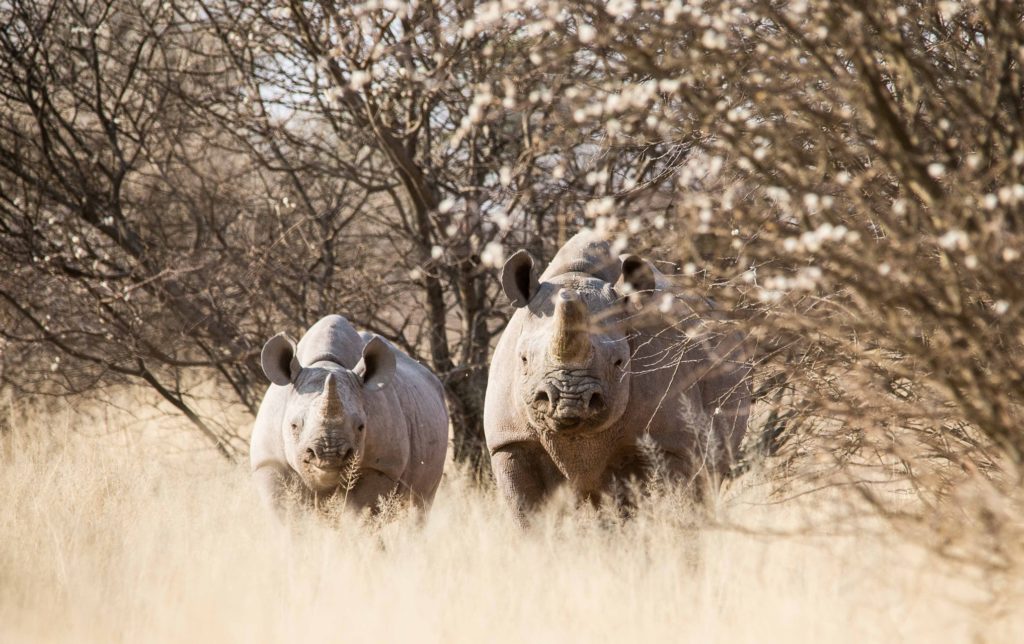
Copyright by Kuzikus Wildlife Reserve
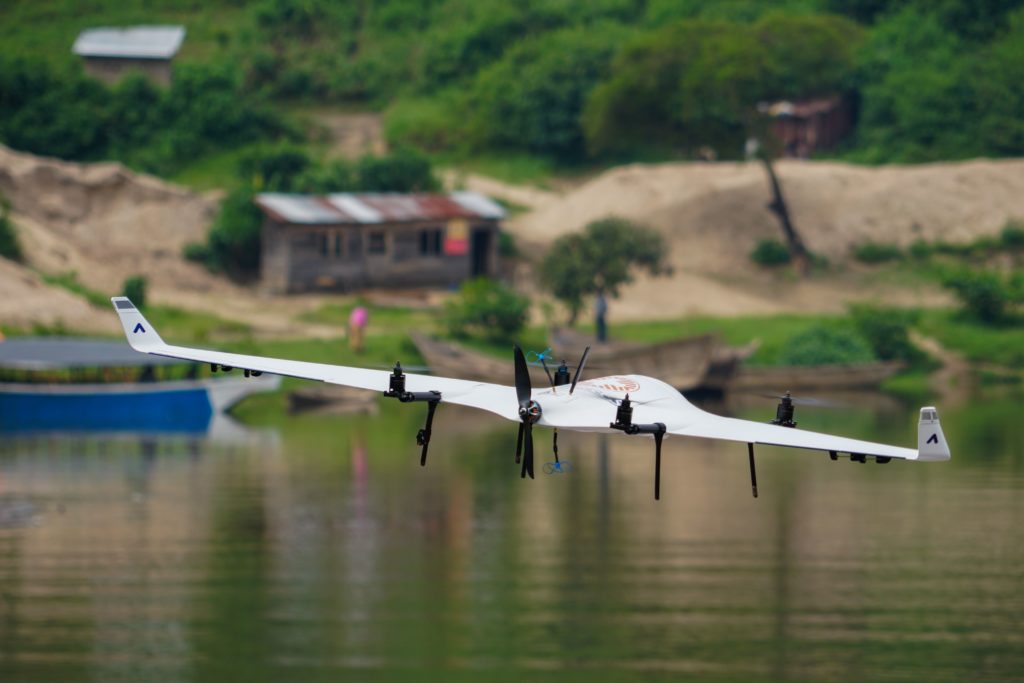
Copyright by Avy
Objectives
Combining multi-modal drone data (e.g., stereovision, 3D sensing, IMUs) into a novel SfM pipeline which explicitly addresses critical configurations to extract accurate terrain reconstructions. The resultant 3D point clouds will then be clustered into (individual) vegetation and ground voxel using supervised and unsupervised machine learning techniques. Critical configurations will be addressed by adding additional constraints to the underlying bundle-adjustment optimization and by fusing sensor readings. The reconstructions will be complemented by a semantic segmentation procedure to identify ground and vegetation geometries (to assess habitat suitability/quality/type) and will be optimized towards state-of-the-art gaming engines enabling GPU accelerated virtual reality rendering for elaborate terrain inspections.
Expected Results
- Scalable terrain reconstruction framework featuring colorized point clouds, mesh models.
- Semantic clusters for habitat analysis.
- Object abstractions (e.g., plant skeletonizations) to support state-of-the-art gaming engines and hardware.
Project Facts
University of Münster (DE).
Professor Benjamin Risse, University of Münster.
Kuzikus African Safaris PTY (NA): Data collection and field evaluation.
AVY (NL): Experimental evaluation using the Avy drone platform.
Get in touch
Contact us on WildDrone@sdu.dk
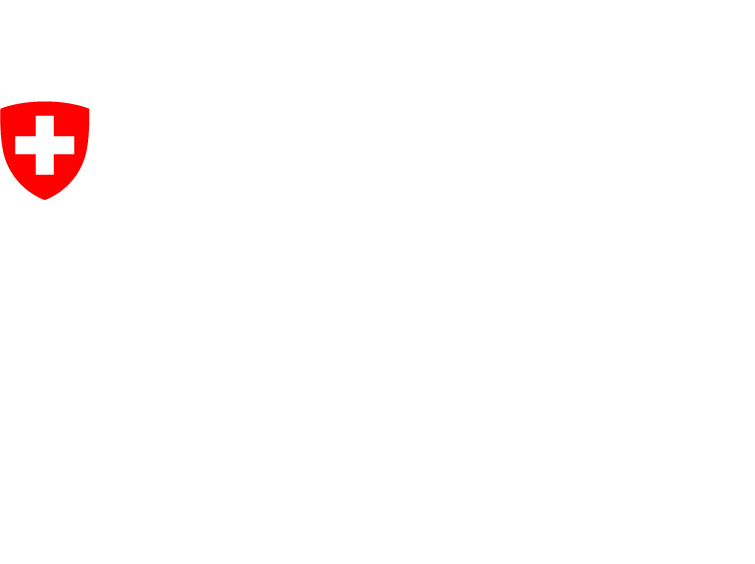
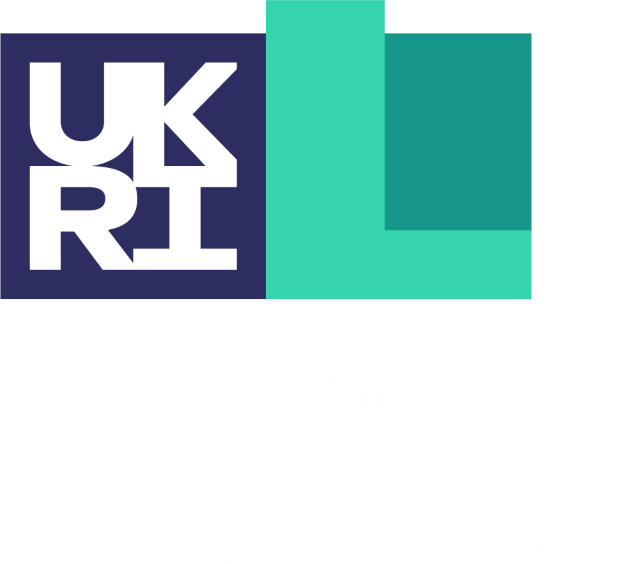
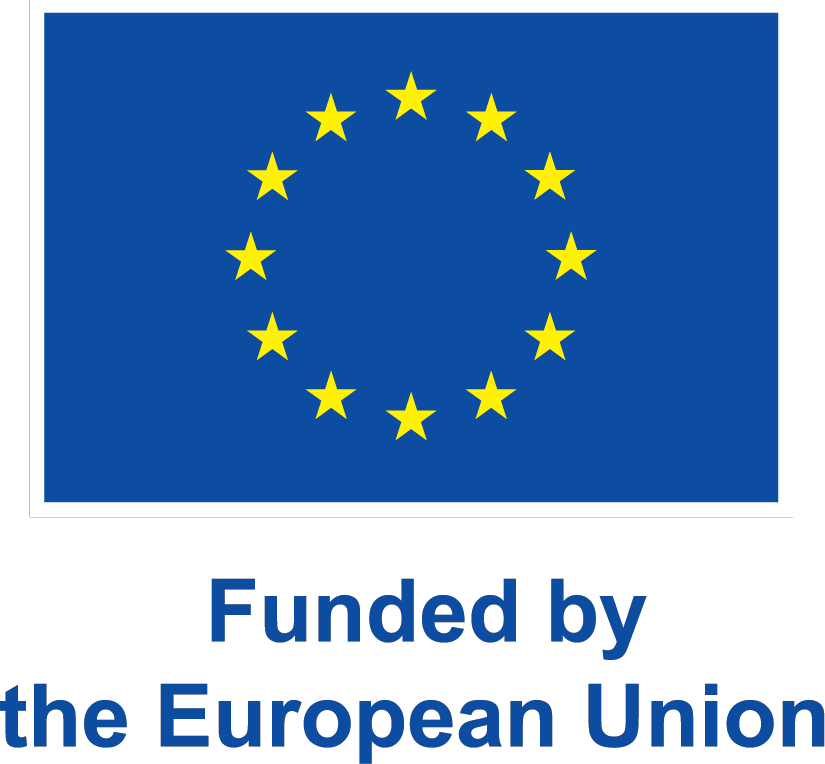
WildDrone is an MSCA Doctoral Network funded by the European Union’s Horizon Europe research and innovation funding programme under the Marie Skłodowska-Curie grant agreement no. 101071224. Views and opinions expressed are those of the author(s) only and do not necessarily reflect those of the European Union or the European Commission. Neither the EU nor the EC can be held responsible for them.