Adaptive Tracking for Detection and Identification
State of the Art
Current drone operations typically consist of flying pre-determined routes capturing data which is analysed post-flight. Some systems allow for manual intervention, but this is often limited by operator experience and the challenges involved in remote operation. They are therefore limited to collecting single viewpoint data and recording imagery passively which has not been optimized for the wildlife in view. The required post-flight analysis can be ambiguous, time consuming, expensive, and laborious.
Innovations and Impact
The close integration of active flight control and planning with onboard computer vision systems. For specific target species, this will use onboard vision data to re-route the aircraft in real-time to enable close-up identification, modelling and analysis of individual animals based on high-resolution sequential images. The drones will be able to actively and dynamically change flight paths to optimize data capture, delivering error-resilient animal recognition and monitoring from the air.
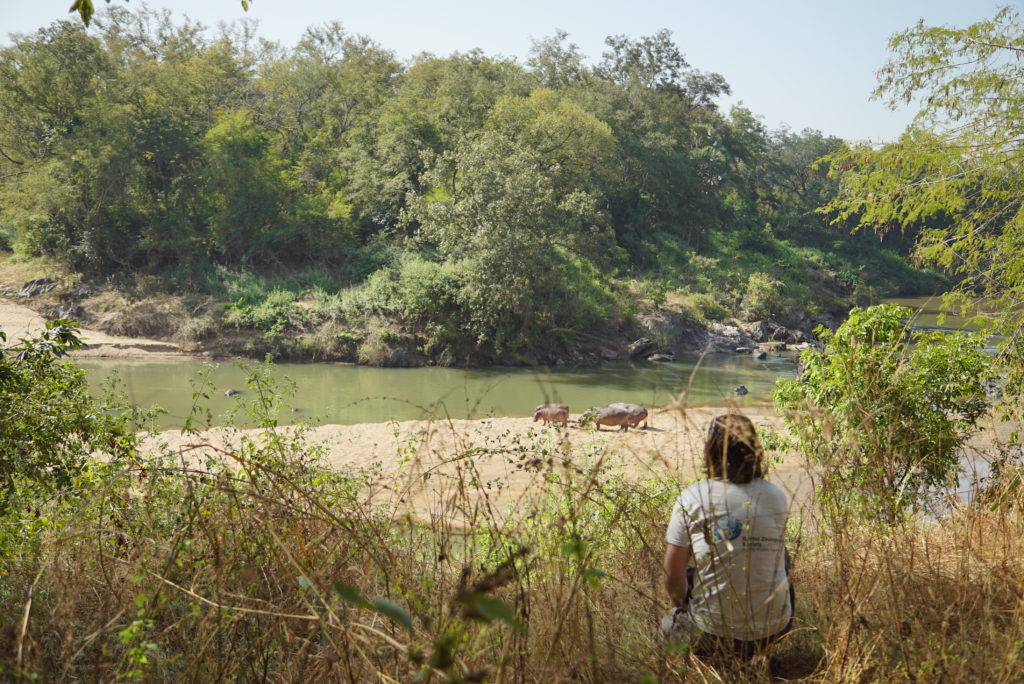
Copyright by University of Bristol
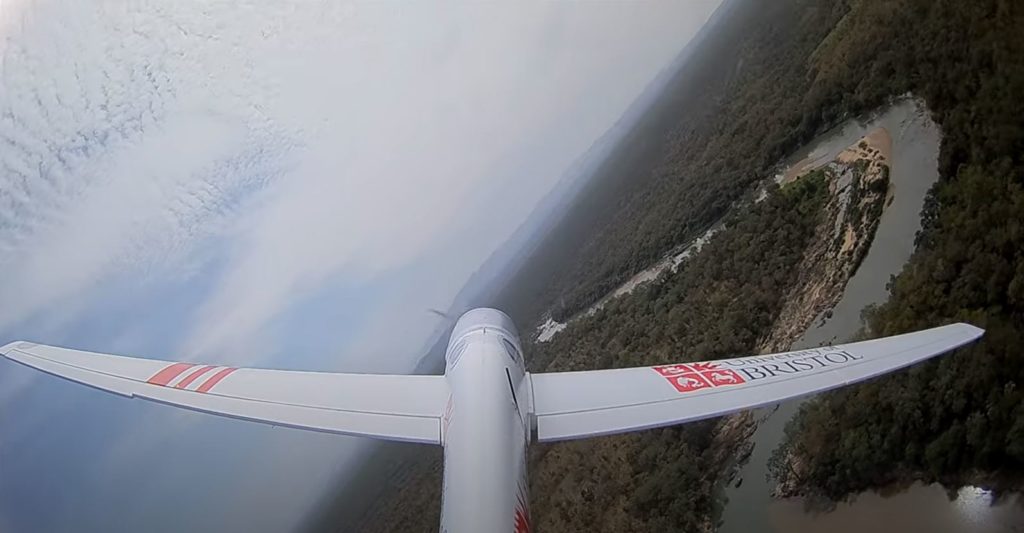
Copyright by University of Bristol
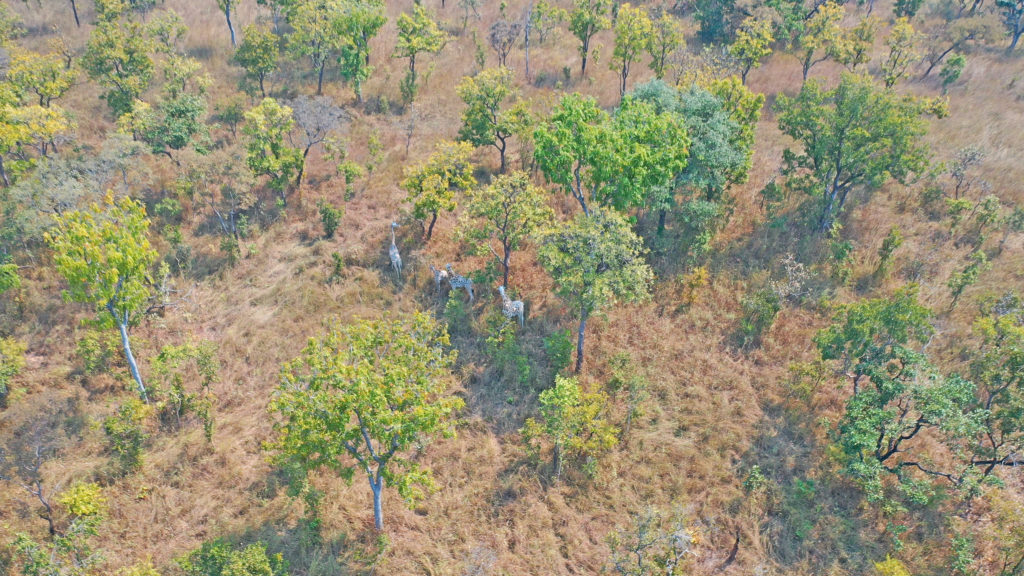
Copyright by University of Bristol
Objectives
The Doctoral Candidate will determine a suitable base architecture for onboard animal detection and identification given onboard GPU constraints and real-time requirements. Single frame detection will be extended to multi-frame species detection and will be linked to navigational goal generation for adaptive tracking. RCN-based open-set recognition solutions, such as metric learning based on multi-view frame sequences will form the basis for this work. Close integration of the aircraft control and vision systems will extend the generation of navigational goals to allow for confirmation of the presence of an individual. Longer term objectives will extend the work to include large group tracking and identification of species behaviour.
Expected Results
The Doctoral Candidate project will create a closely integrated deep learning system capable of producing achievable navigational goals to verify ambiguous candidate detections from multiple viewing angles. To increase detection robustness, entire frame sequences will be utilised to determine species candidates. The onboard system will actively seek suitable high-quality oblique observational positions for close-ups to improve acquisition until identification can be reliably confirmed.
Project Facts
Planned Secondments: University of Münster (Germany); real-time computer vision. EPFL (Switzerland); open-set recognition solution.
Principal Supervisor: Tilo Burghardt – Senior Lecturer, University of Bristol.
Academic Supervisor: Professor Thomas Richardson, University of Bristol.
Main hosting site: University of Bristol (United Kingdom).
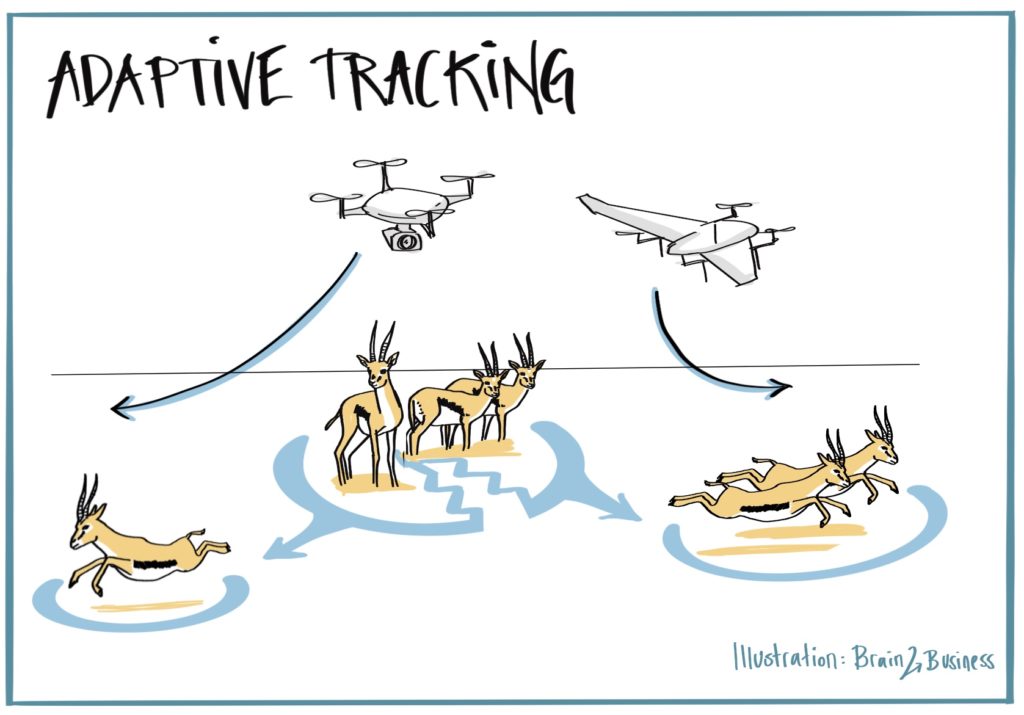
Illustration of DC12 – Adaptive Tracking project
More about the Themes
Get in touch
Contact us on WildDrone@sdu.dk
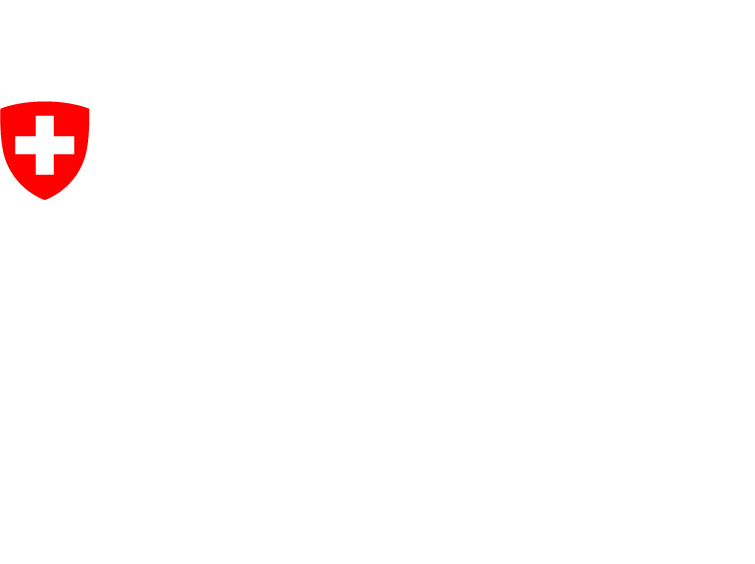
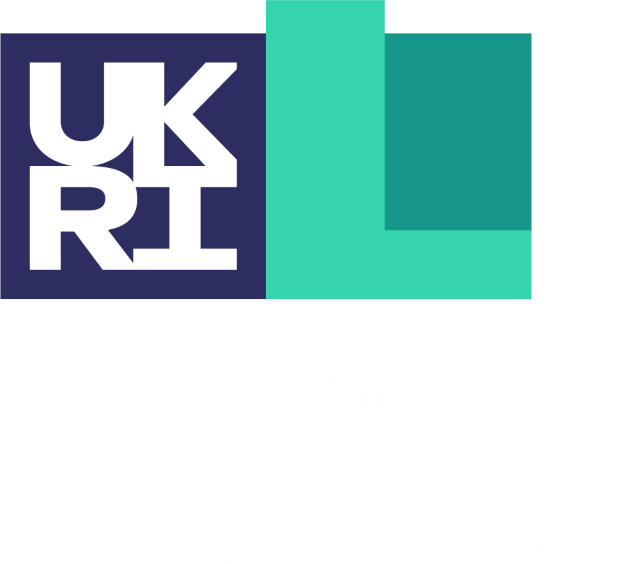
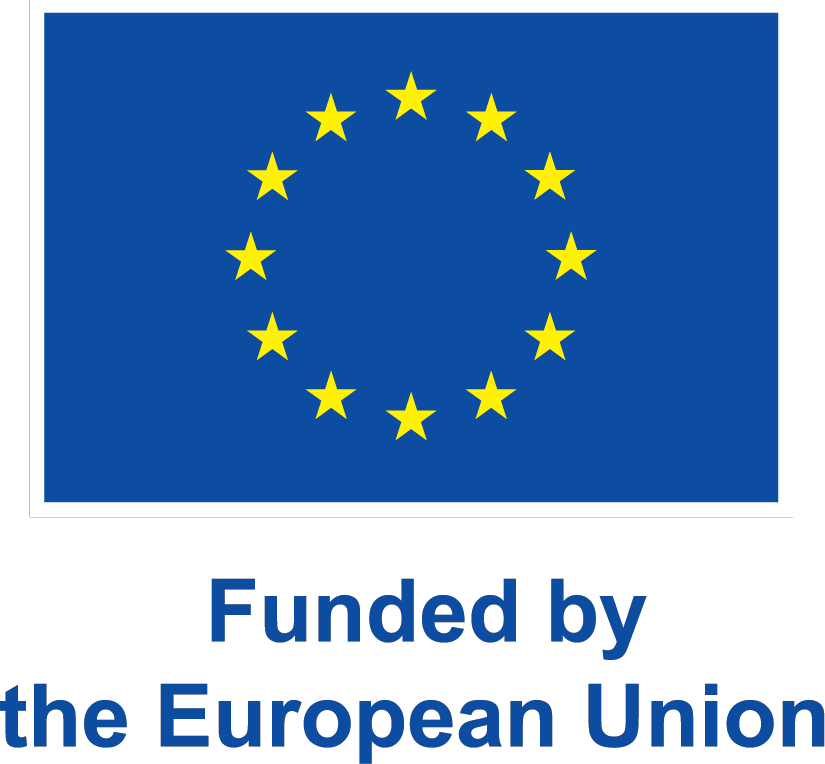
WildDrone is an MSCA Doctoral Network funded by the European Union’s Horizon Europe research and innovation funding programme under the Marie Skłodowska-Curie grant agreement no. 101071224. Views and opinions expressed are those of the author(s) only and do not necessarily reflect those of the European Union or the European Commission. Neither the EU nor the EC can be held responsible for them.