Theme 1
Automated Wildlife Tracking for Conservation Practice
The doctoral projects under Theme 1 have two main objectives.
Application of Drones as a Tool for Predicting and Preventing Conflict Between Wildlife and Humans
As human populations expand into natural areas, conflicts between people and animals increase. Human-initiated conflicts, such as poaching and noise pollution, are major threats to species around the world[1],[2],[3]. Anti-poaching efforts are limited by the ability of rangers to efficiently patrol vast protected areas to detect and apprehend poachers[4],[5]. Anthropogenic marine activities, such as drilling and construction, can negatively impact marine fauna over vast areas3. Other conflicts, such as livestock predation and wildlife-mediated disease transmission negatively impact human livelihoods, engender negative attitudes toward wildlife, and prompt retaliatory killings[6],[7]. Automated monitoring of the movement and behaviour of relevant animal groups could allow conservation practitioners to mitigate such conflicts, e.g., by rapidly detecting poachers based on wildlife behavioural responses to human presence (DC3 – Predator-prey Response), ceasing construction work when sensitive wildlife is present (DC4 – Coastal Monitoring), or redirecting grazing livestock to avoid predators and disease vectors (DC1 – Livestock-wildlife Interaction).
Integration of Drone Systems with Bio-logging Technologies to Link Animal Behaviour with Physical and Ecological Context
Recent advances in animal-mounted GNSS tags have revolutionized ecologists’ ability to collect movement data on a wide range of animal species as they traverse landscapes, continents, and hemispheres[1]. To interpret this data, researchers need to understand the environmental and social context of animal movement behaviour. Secondary sensors, such as cameras, altimeters and thermometers can provide contextual data but are often heavy and resource-intensive, and therefore unsuitable for mounting on many wildlife species[2]. Drones can be flexibly deployed to gather data on terrain, land use, and environmental factors such as wind and thermal uplifts, as well as on animals themselves and their interactions[3],[4]. Drones have previously been combined with animal tracking to document environmental conditions along avian foraging paths[5],[6], compare the quality of ungulate foraging habitats[7], and monitor deforestation in primate home ranges[8]. So far, applications have relied heavily on visual sensors, and researchers have manually converted animal movement paths into flight missions. Incorporating a wider range of sensors into drone-based missions and developing automated means of integrating bio-logging tags and drone operating systems will allow for more efficient collection of a wider range of relevant contextual data, including the behaviour of prey in response to tagged predators (DC3 – Predator-prey Response) and the air conditions and landscape cues that affect the flight paths of migratory birds (DC2 – Migration Analysis). Moreover, monitoring of specific species’ populations and health is an urgent need across Europe and Africa, including detailed real-time census of coastal birds and marine mammals in the Wadden Sea area (DC8 – Real-time Census), but no automated solutions are available.
References
Objective 1
[1] M.Linkie, D.J. Martyr, J.Holden, A.Yanuar. 2003. Habitat destruction and poaching threating the Sumatran tiger in Kerinci Seblat National Park, Sumatra. Oryx, 37, 41-48.
[2] S.M.Ferreira, C.Greaver, G.A.Knight, M.H.Knight, I.P.J.Smith, D.Pienaar. 2015. Disruption of rhino demography by poachers may lead to population declines in Kruger National Park, South Africa. PLoS ONE, 10, e0127783.
[3] L.S.Weilgart. 2007. The impacts of anthropogenic noise on cetaceans and implications for management. Can J Zool, 85, 1091-1116.
[4] M. Mulero-Pázmány, R. Stolper, L.D. van Essen, J.J. Negro, T. Sassen. 2014. Remotely piloted aircraft systems as a rhinoceros anti-poaching tool in Africa. PLoS ONE, 9, e83873.
[5] H.J. de Knegt, J.A.J. Eikelboom, F. van Langevelde, W.F. Spruyt, H.H.T. Prins. 2021. Timely poacher detection and localization using sentinel animal movement. Sci Rep-UK, 11, 4596.
[6] R.Woodroffe, S.Thirgood, A.Rabinowitz. (Eds). 2005. People and Wildlife, Conflict or Co-existence? (Conservation Biology). Cambridge University Press.
[7]A.Treves, R.B.Wallace, L.Naughton-Treves, A.Morales,. 2007. Co-Managing human-wildlife conflicts: a review. Human Dimen. of Wildlife, 11, 383-396.
Objective 2
[1] R.Kays, M.C.Crofoot, W.Jetz, M.Wikelski. 2015. Terrestrial animal tracking as an eye on life and planet. Science, 348, aaa2478.
[2] H.J.Williams, L.A.Taylor, S.Benhamou, A.I.Bijleveld, T.A.Clay, S.de Grissac, U.Demsar, H.M.English, N.Franconi, A.Gómez-Laich, R.C.Griffiths, W.P.Kay, J.M.Morales, J.R.Potts, K.F.Rogerson, C.Rutz, A.Spelt, A.M.Trevail, R.P.Wilson, L.Börger. 2020. Optimizing the use of biologgers for movement ecology research. J Anim Ecol, 89, 186-206.
[3] K.Anderson, K.J.Gaston. 2013. Lightweight unmanned aerial vehicles will revolutionize spatial ecology. Front Ecol Environ, 11, 138-146.
[4] J.M.Graving, D.Chae, H.Naik, L.Li, B.Koger, B.R.Costelloe, I.D. Couzin. 2019. DeepPoseKit, a software toolkit for fast and robust animal pose estimation using deep learning. eLife, 8, e47994.
[5] A.Rodríguez, J.J.Negro, M.Mulero, C.Rodríguez, J.Hernández-Pliego, J.Bustamente. 2012. The eye in the sky: combined use of unmanned aerial systems and GPS data loggers for ecological research and conservation of small birds. PLOS ONE, 7, e50336.
[6] C.C.Wilmers, B.Nickel, C.M.Bryce, J.A.Smith, R.E.Wheat, V.Yovovich. 2015. The golden age of bio-logging: how animal-borne sensors are advancing the frontiers of ecology. Ecology, 96, 1741-1753.
[7] A.K.Scheiger, M.Schütz, P.Anderwald, M.E.Schaepman, M.Kneubühler, R.Haller, A.C.Risch. 2015. Foraging ecology of three sympatric ungulate species: behavioral and resource maps indicate differences between chamois, ibex and red deer. Mov Ecol, 3, 6.
[8] D.J.Stark, I.P.Vaughan, L.J.Evans, H.Kler, B.Goossens. 2017. Combining drones and satellite tracking as an effective tool for informing policy change in riparian habitats: a proboscis monkey case study. Remote Sensing in Ecology and Conservation, 4, 44-52.
Get in touch
Contact us on WildDrone@sdu.dk
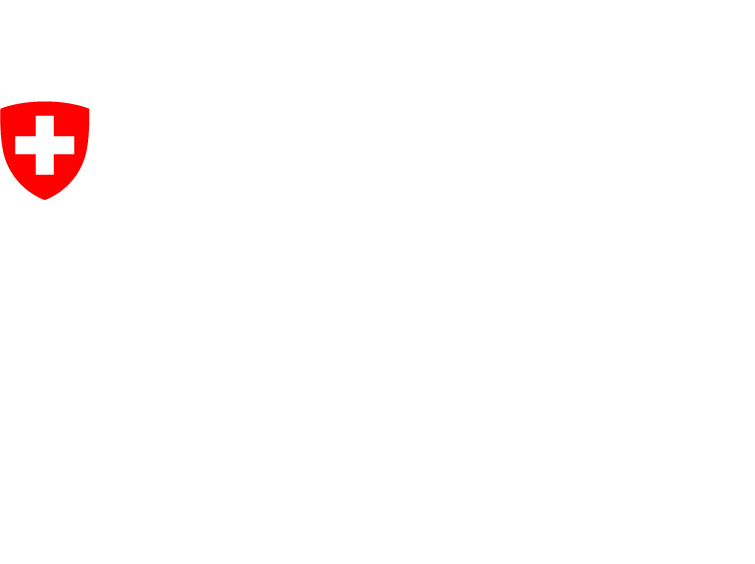
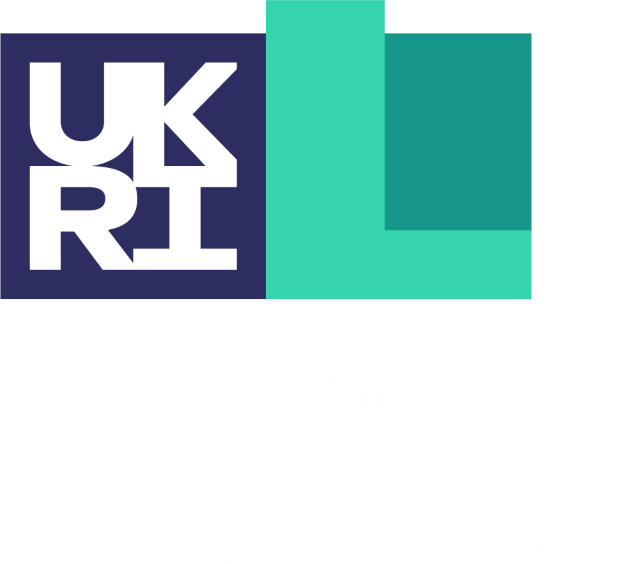
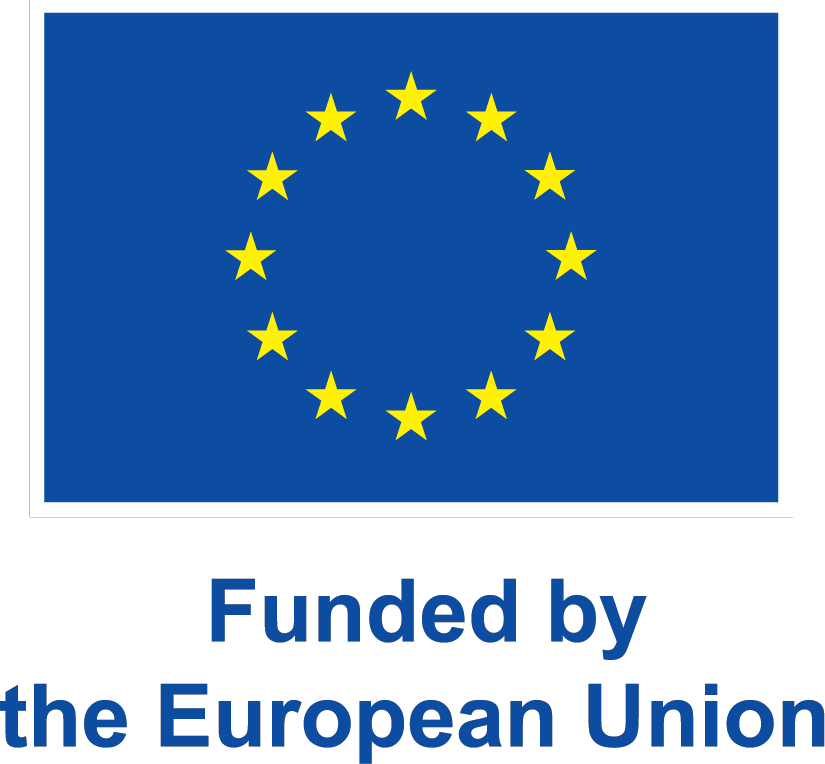
WildDrone is an MSCA Doctoral Network funded by the European Union’s Horizon Europe research and innovation funding programme under the Marie Skłodowska-Curie grant agreement no. 101071224. Views and opinions expressed are those of the author(s) only and do not necessarily reflect those of the European Union or the European Commission. Neither the EU nor the EC can be held responsible for them.