Individual Characteristics
Detecting Posture, Metrics and Biometrics of Animals from Drone Data
State of the Art
Data fusion and real-time processing often perform poorly when applied in non-controlled outdoor environments and on moving platforms. Moreover, the detection of wildlife posture and metrics suffer of ill-pose sensor positioning, occlusions, low-resolution data, diversity of animals’ appearances, and dynamics. Various methods were designed for laboratory experiments and fail in real wild environments.
Innovations and Impact
Design and realization of a drone-based real-time method, based on data fusion and SLAM solutions, to detect animals, track them, and derive metrics. This approach will also leverage on re-routing capabilities to enable close-up views and higher-resolution data acquisition. The solution will be deployable also in other sectors (surveillance, rescue, and search, etc.) enlarging the impact of the work.
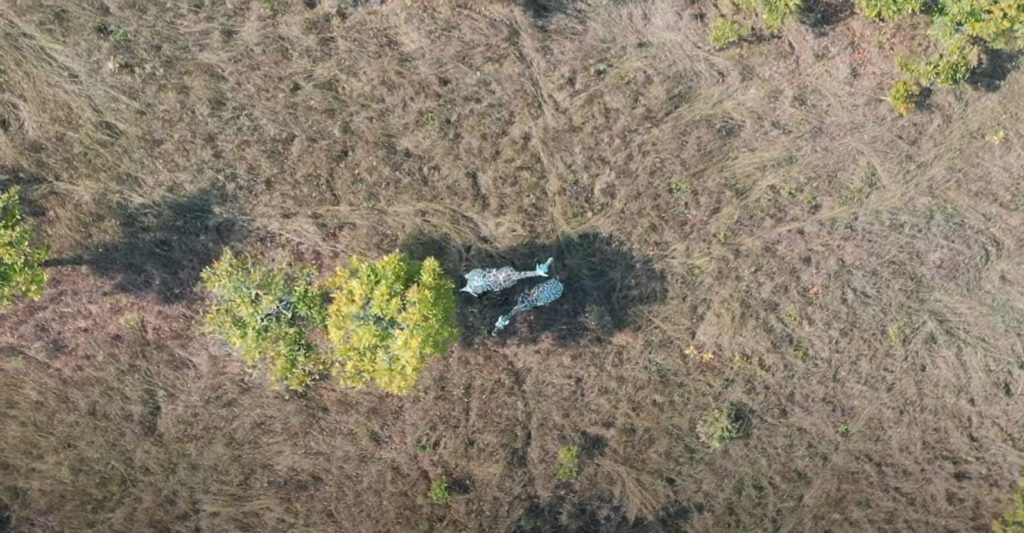
Copyright by University of Bristol
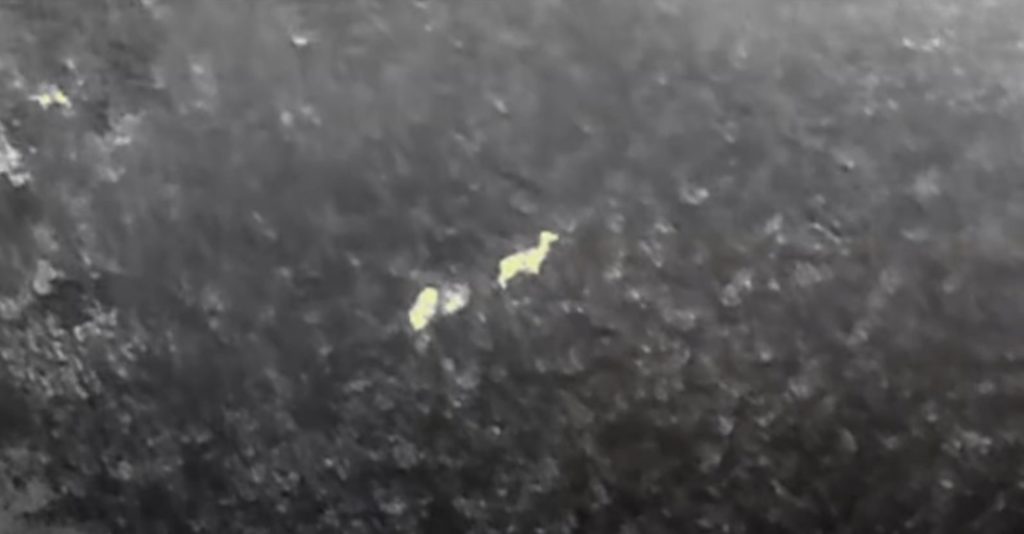
Copyright by University of Bristol
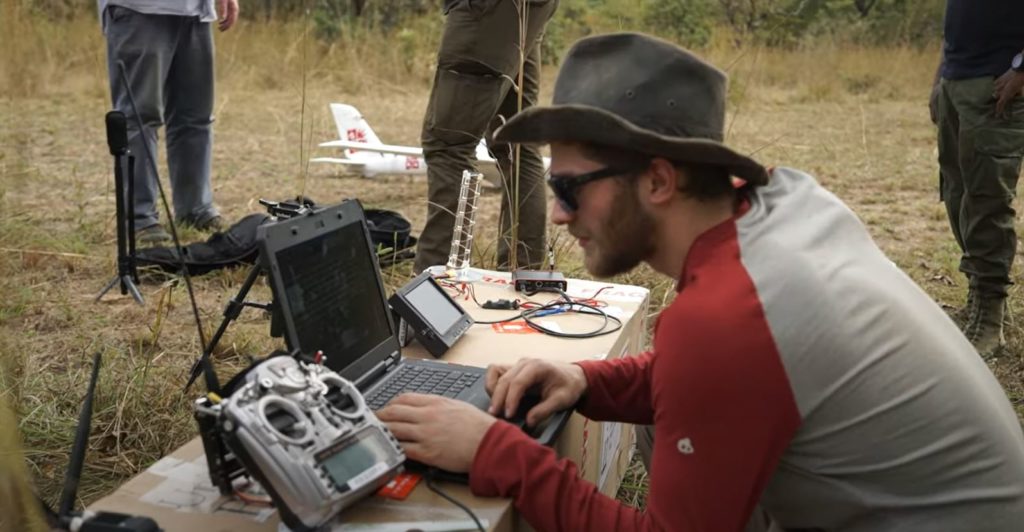
Copyright by University of Bristol
Objectives
DC9 will investigate and develop new solutions for autonomous planning of drone flights and automated scene interpretation based on AI methods. Firstly, the research will realize an advanced planning tool to optimize flight resources (with respect to batteries, time, etc.) and achieve safe and autonomous surveying of wild areas with animals. Secondly, analysing, processing, and fusing all data collected by the onboard sensors (cameras, LiDAR, IMU/GNSS), it will help to deliver innovative algorithms to track and recognize animals as well as provide 3D measurements. Sensor data will be processed with SLAM-based methods coupled with machine learning techniques to support identification, semantic segmentation, and 3D reconstruction. All processing will take place onboard within a Jetson or Raspberry device. The developed solutions, tested and validated using imagery acquired in partner wildlife parks, will facilitate to detect postures and biometrics useful for monitoring and conservation purposes.
Expected Results
- Onboard SLAM-based methods for wildlife tracking and identification (instance segmentation).
- Real-time 3D mapping of wildlife and surveyed scene.
- Derivation of posture and biometrics.
- Integration with flight planning / re-routing solutions aided by SLAM-based methods.
Project Facts
Fondazione Bruno Kessler (IT).
The Ph.D. will be awarded by University of Münster (DE).
Professor Fabio Remondino and Dr Andrea Micheli, Bruno Kessler Foundation (IT).
Professor Benjamin Risse, University of Münster.
University of Bristol (UK): Flight planning, flight dynamics.
University of Münster (DE): Tracking, segmentation, SLAM.
Get in touch
Contact us on WildDrone@sdu.dk
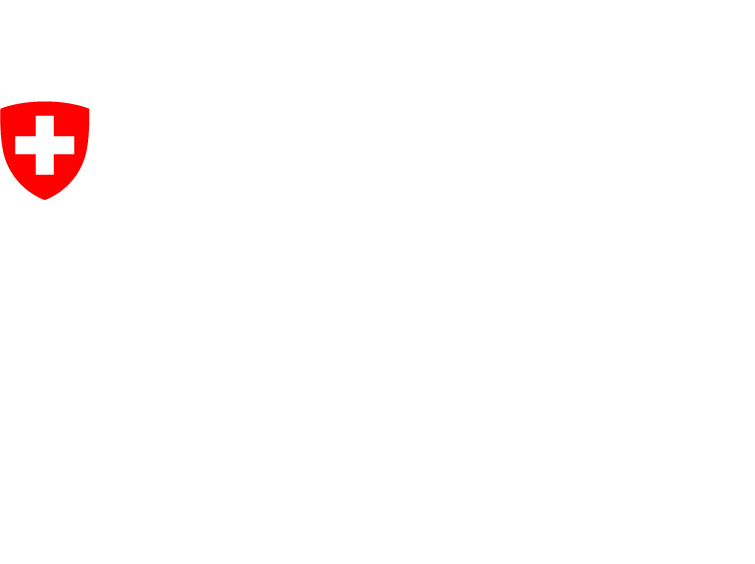
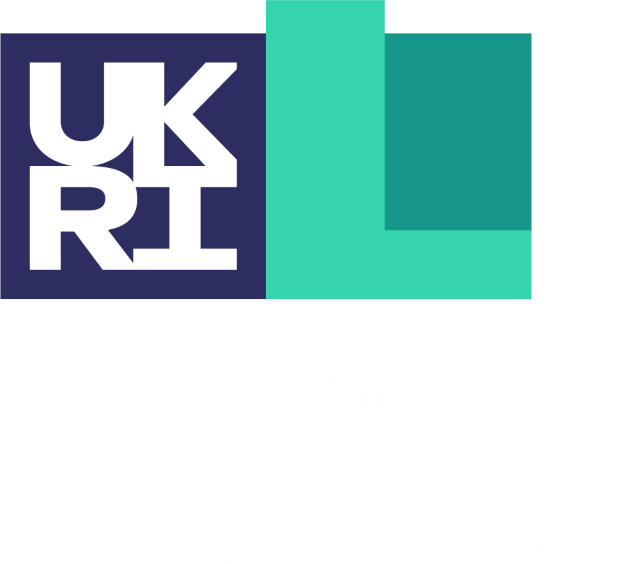
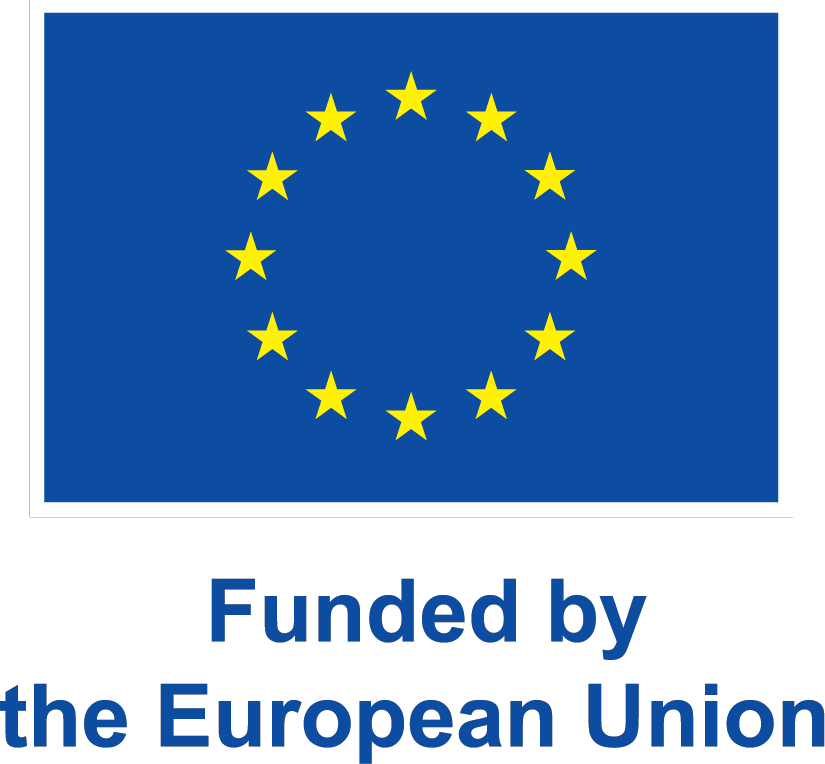
WildDrone is an MSCA Doctoral Network funded by the European Union’s Horizon Europe research and innovation funding programme under the Marie Skłodowska-Curie grant agreement no. 101071224. Views and opinions expressed are those of the author(s) only and do not necessarily reflect those of the European Union or the European Commission. Neither the EU nor the EC can be held responsible for them.